Solving the Data Conundrum in Healthcare
Barriers to Innovation in Healthcare: The first of a three part series.
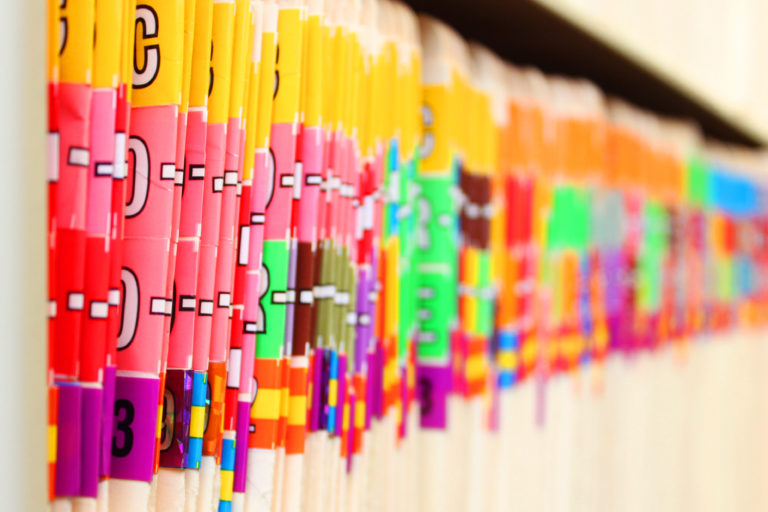
Healthcare’s transition to a highly data-centric environment, requires greater innovation and technology than ever before. While healthcare has always been a data-driven field (through laboratory tests, imaging exams, vital signs, and other clinical tests), we have recently made the jump from having many analog repositories (paper charts, etc.) to a full digital data repository environment (EHR, PACS, etc.). This major transition introduced new obstacles in the areas of data aggregation, data normalization, data processing, and data output analytics. In an analog world, the issue of data management did not exist due to the disparate nature of a paper-centric environment. Each hospital was responsible for the management of its own data in the form of charts and paper forms; so the urgency or need to to achieve high volume processing did not exist (the inherent limitations were essentially universal). With healthcare costs continually rising and life expectancy rates ultimately increasing in the face of expensive chronic disease conditions, it is imperative we leverage innovation and technology through agile means to solve the required data processing problems of today and for the future.
The first step to solving the data conundrum is to identify the barriers present. In the case of data management for healthcare, there are many barriers that impede innovation and ultimately impact quality. This leaves an institution facing a situation in which resource requirements are not acceptable and costs may be prohibitive. There are five major barriers that exist in some form or other and will vary across institutions: 1) unstructured data, 2) data aggregation, 3) data entry, 4) technology infrastructure, and 5) meaningful insights.
Unstructured Data
One major set-back to an organization’s potential success is the issue of unstructured data. Approximately 85 percent of healthcare records exist in the form of unstructured data. This means an institution may be “data-rich,” but is essentially “information-poor” due to the lack of structuring of its own data. Extensive data sets are buried in repositories without any discernible structure, tagging, or sorting that would allow for easy retrieval and query functions. One may be looking for an instance of “nodule” throughout a patient record, but a query may return findings associated with disparate body regions, operative notes, imaging exams, etc. Adding appropriate structure to the data set, a query for nodule could prompt further specification as to pulmonary nodule found on chest CT imaging, and even further allow for specifying location (i.e. right lung, lower lobe). Data structure then becomes more valuable.
Data Aggregation
Even if an institution is able to add structure to its data, that still does not solve the issue of data aggregation. As noted above, large volumes of data are commonly stored or harbored in multiple disparate data repositories. Imaging is stored on PACS, pathology may be in the form of scanned reports, cardiology exams in C-PACS, radiology information in RIS, consult notes in EHR, clinic notes in a remote clinic EHR, and other data elements may still exist in a separate HIS. Tying it all together becomes tedious and resource intensive. An organization is burdened with connecting all disparate sources in a clean and efficient manner, while not complicating its data structure through redundancy or missing elements. Ultimately, an ideal solution is one that is “patient-centric.” Patient-centricity is only achievable through efficient and accurate aggregation of data, functioning as a single repository. An example would be a primary physician reviewing the procedure and lab history of a patient that has undergone various procedures at different sites and through different modalities. Regardless of where the data elements exist, they should be available and accessible based on patient ownership. Data aggregation thus adds value to a healthcare organization.
Data Entry
Data aggregation and data structuring barriers alone are sufficiently burdensome. Add to that the common requirement for data registry entry, and an institution can easily become overwhelmed. Today, there is a constant need for data submission into large national data registries. Efficiency is usually at a premium. Resource requirements can cost multiple FTEs. The impact is significant and real. If data is not submitted to various registries, reimbursement may be affected, ultimately affecting an institutions bottom line. Many institutions rely on manual entry to achieve data registry submission. It is not uncommon to see a scenario in which a nurse navigator is tasked with owning a spreadsheet file that requires constant manual data input and manual submission to a registry. This consumes substantial time for a single individual, so much so that a separate navigator is required simply to allow for service line or program growth. Complicate that scenario by adding a separate program into a service line, and now another FTE resource is required. The resource requirements are obvious, but more unfortunate is the impact created by separation of programs within a single service line. An example would be a single Oncology service line that benefits from a single oncology nurse navigator having the platform and resources to be able to manage data submission for lung cancer screening, mammography screening, and colonoscopy screening. Data submission would then only require a small portion of time allowing for program enhancements through physician outreach and education. Program growth is then feasible and even embraced.
Technology Infrastructure
Without the appropriate technology infrastructure, none of the aforementioned obstacles may be overcome. The focus lies squarely on technology and innovation. An organization needs a sound technology infrastructure in order to benefit from the innovation tools that are available. Structuring of data may require the establishment of server-level data sorting algorithms in order to process data elements, which then allows for proper sorting into a database format. Database environments have to be robust and scalable. An organization needs to plan its database environment to accommodate for a 500,000 patient population just as it would for a 100 million patient population. Interfaces to the the disparate data repositories have to be established. Different repositories require different data feeds or “calls” in order to access specific data elements. The centralization of data frequently relies on the creation of APIs (application programming interfaces) in order to share data, usually back and forth. After the appropriate APIs and interfaces are established, data warehousing must be considered. Data warehouses may be large physical and/or virtual sites in which the data will reside. Leveraging cloud-based solutions ultimately allows for endless scalability and exceptional safety through unparalleled redundancy. Some organizations may rely on physical locations to store their data, with astronomical cost attributed to the maintenance of server health and data redundancy. An example may be a large multi-center organization looking to centralize its numerous disparate data repositories into a central unified database, but it is impeded by the physical data storage requirements and server maintenance costs. Sacrifices are then made, usually in the form of time and money or through restriction of resources that would otherwise allow for large scale program growth.
Meaningful Insights
An organization may have achieved solutions to the above barriers, but one problem may still exist that potentially could add the most value to institution. Data must be analyzed and utilized to generate meaningful insights. Once data is cleaned, sorted, aggregated, and readily accessible, it still does not solve the problem with analytics. How does an organization gain knowledge and insight about its patient population? This occurs through trend analysis and operational assessment. Data analysis may uncover unknown any previously unseen obstacles at the organizational level that prevented patient access to care. Analytics may reveal that an organization’s patient population is at higher risk for various disease processes relative to other areas of the country, thus necessitating the creation of a new separate screening initiative or service line. An example would be an organization that realizes it has a high incidence of non-smoking related lung malignancy. Awareness is increased and a new program is started to further study risk factors associated with the malignancy. Research is generated and published, and access to screening or testing is increased for patients in the area. Ultimately, knowledge is gained which empowers an institution and, more importantly, improves patient care.
Having identified the existing barriers to solving the data conundrum, innovative solutions are becoming more readily available as the needs continue to be refined. Read part 2 of 3 of this series on innovation and technologies as solutions.
About Thynk Health
The Thynk Health platform optimizes data-driven workflows and provides operational and clinical analytics for lung cancer screening programs and other quality initiatives.