Changing the Way Radiologists Performance is Assessed with the help of Machine Learning and Natural Language Processing
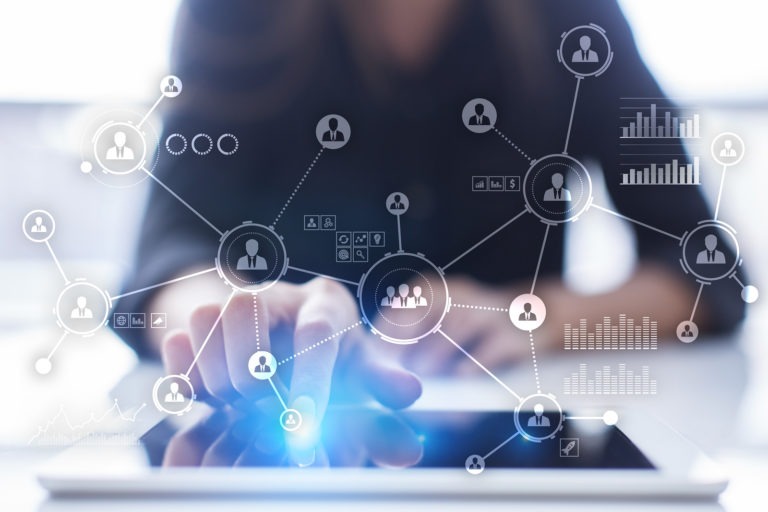
Radiologists quality evaluation programs are highly variable and rarely lead to improved patient care. Some organizations like the American College of Radiology have substantially elevated the topic by creating frameworks and data repositories to collect information on radiologists to benchmark performance across the nation. MIPS (formally PQRS) developed measures that are radiology specific in an attempt to use a CPT code-based structure to determine reported language within the report, typically using manual abstraction approaches. Registry approaches such as these provide a useful framework but can be challenging to track, as large organizations have limited resources to track only a few of these questionably valuable measures.
So how do we get an accurate picture of radiologists’ quality of imaging interpretation without adding unreasonable amounts of analytics support staff to conduct unsustainable degrees of manual abstraction? One approach is to utilize NLP and other ML techniques that will constantly ‘read’ radiology reports, with contextual understanding, while applying the results against the patient’s history and known guidelines to determine standardized reporting practices as well as appropriate follow-up management suggestions.
By leveraging these techniques, Thynk Health is seeing programs accelerate their standardized reporting practices based on monthly analytics feedback reports to radiologists. It is not uncommon for the Thynk Health NLP solution to uncover highly inconsistent reporting language around the same findings amongst radiologists; even in the same group. Powered with very detailed analytics reports, operational input and reporting guideline suggestions – the groups, realize very rapid improvement to achieve close to 100 percent consistency in reporting language based on the latest follow-up management and findings descriptor recommendations. Although the primary intent of our solution is the management of more extensive programs, organizations have found the added benefit of using our NLP technologies drives standardization in operations and reporting for radiology programs.
While NLP remains a required key in analyzing radiology reporting quality, computer vision of the images themselves, for comparison to those reported findings will offer the most power. An example use case registry could include all of an organization’s body CTs being processed for renal masses using algorithms that can visually detect renal mass size and characteristic compared against discrete language extracted from the radiologist’s report using NLP. These language elements would include description language and follow-up management details. Human validation of outlier cases will still be required as these technologies are not perfect.
The holy grail, will of course, be in the point of care processes that will ultimately come from ML and NLP providing real-time guidance to the radiologist to infuse quality from the beginning. An accurate and easy-to-use interface that would allow for wide-scale radiologists adoption into daily workflows is at least five to ten years away. In the interim, good quality initiatives can indeed be achieved with the machine learning and natural language processing technologies available on the Thynk Health platform.
About Thynk Health
The Thynk Health platform optimizes data-driven workflows and provides operational and clinical analytics for lung cancer screening programs and other quality initiatives.